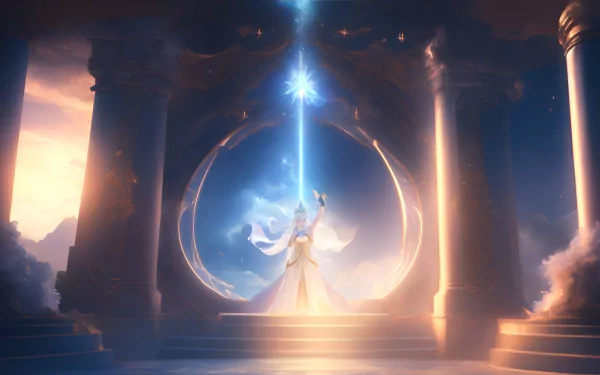
Adeeplearning-basednoisecorrectionmethodforlight-fieldfluorescencemicroscopy
Light-field microscopy (LFM) enables rapid volumetric imaging through single-frame acquisition and fast 3D reconstruction algorithms. The high speed and low phototoxicity of LFM make it highly suitable for real-time 3D fluorescence imaging, such as studies of neural activity monitoring and blood flow analysis. However, in vivo fluorescence imaging scenarios, the light intensity needs to be reduced as much as possible to achieve longer-term observations. The resulting low signal-to-noise ratio (SNR) caused by reduced light intensity significantly degrades the quality of 3D reconstruction in LFM. Existing deep learning-based methods struggle to incorporate the structured intensity distribution and noise characteristics inherent to LFM data, often leading to artifacts and uneven energy distributions. To address these challenges, we propose the denoise-weighted view-channel-depth (DNW-VCD) network, integrating a two-step noise model and energy weight matrix into an LFM reconstruction framework. Additionally, we developed an attenuator-induced imaging system for dual-SNR image acquisition to validate DNW-VCD's performance. Experimental results our method achieves artifact-reduced, real-time 3D imaging with isotropic resolution and lower phototoxicity, as verified through imaging of fluorescent beads, algae, and zebrafish heart.
相关推荐