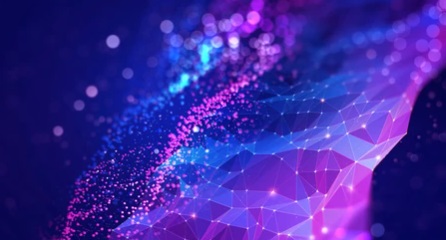
AdversarialExampleBasedFingerprintingforRobustCopyrightProtectioninSplitLearning
Currently, deep learning models are easily exposed to data leakage risks. As a distributed model, Split Learning thus emerged as a solution to address this issue. The model is splitted to avoid data uploading to the server and reduce computing requirements while ensuring data privacy and security. However, the transmission of data between clients and server creates a potential vulnerability. In particular, model is vulnerable to intellectual property (IP) infringement such as piracy. Alarmingly, a dedicated copyright protection framework tailored for Split Learning models is still lacking. To this end, we propose the first copyright protection scheme for Split Learning model, leveraging fingerprint to ensure effective and robust copyright protection. The proposed method first generates a set of specifically designed adversarial examples. Then, we select those examples that would induce misclassifications to form the fingerprint set. These adversarial examples are embedded as fingerprints into the model during the training process. Exhaustive experiments highlight the effectiveness of the scheme. This is demonstrated by a remarkable fingerprint verification success rate (FVSR) of 100% on MNIST, 98% on CIFAR-10, and 100% on ImageNet, respectively. Meanwhile, the model's accuracy only decreases slightly, indicating that the embedded fingerprints do not compromise model performance. Even under label inference attack, our approach consistently achieves a high fingerprint verification success rate that ensures robust verification.
相关推荐