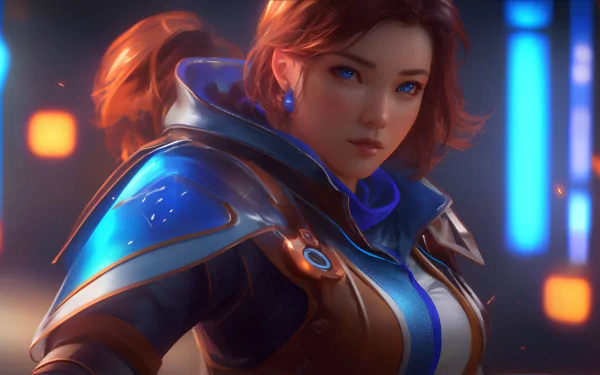
FromStarstoMolecules:AIGuidedDevice-AgnosticSuper-ResolutionImaging
Super-resolution imaging has revolutionized the study of systems ranging from molecular structures to distant galaxies. However, existing deep-learning-based methods require extensive calibration and retraining for each imaging setup, limiting their practical deployment. We introduce a device-agnostic deep-learning framework for super-resolution imaging of point-like emitters that eliminates the need for calibration data or explicit knowledge of optical system parameters. Our model is trained on a diverse, numerically simulated dataset encompassing a broad range of imaging conditions, enabling robust generalization across different optical setups. Once trained, it reconstructs super-resolved images directly from a single resolution-limited camera frame with superior accuracy and computational efficiency compared to conventional methods. We experimentally validate our approach using a custom-built microscopy setup with ground truth emitter positions and demonstrate its versatility on astronomical and single-molecule localization microscopy datasets, achieving unprecedented resolution without prior information. Our findings establish a pathway toward universal, calibration-free super-resolution imaging, expanding its applicability across scientific disciplines.
相关推荐