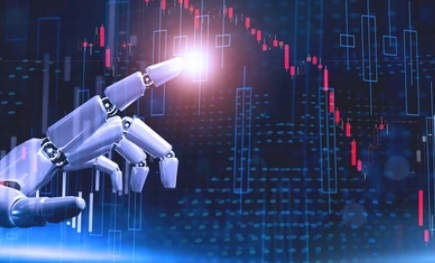
AdaptiveBiasedUserSchedulingforHeterogeneousWirelessFederateLearningNetwork
Federated Learning (FL) has revolutionized collaborative model training in distributed networks, prioritizing data privacy and communication efficiency. This paper investigates efficient deployment of FL in wireless heterogeneous networks, focusing on strategies to accelerate convergence despite stragglers. The primary objective is to minimize long-term convergence wall-clock time through optimized user scheduling and resource allocation. While stragglers may introduce delays in a single round, their inclusion can expedite subsequent rounds, particularly when they possess critical information. Moreover, balancing single-round duration with the number of cumulative rounds, compounded by dynamic training and transmission conditions, necessitates a novel approach beyond conventional optimization solutions. To tackle these challenges, convergence analysis with respect to adaptive and biased scheduling is derived. Then, by factoring in real-time system and statistical information, including diverse energy constraints and users' energy harvesting capabilities, a deep reinforcement learning approach, empowered by proximal policy optimization, is employed to adaptively select user sets. For the scheduled users, Lagrangian decomposition is applied to optimize local resource utilization, further enhancing system efficiency. Simulation results validate the effectiveness and robustness of the proposed framework for various FL tasks, demonstrating reduced task time compared to existing benchmarks under various settings.
相关推荐
热门文章
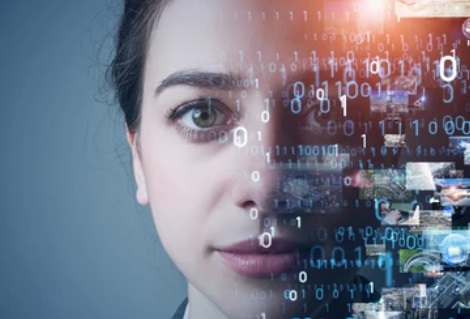