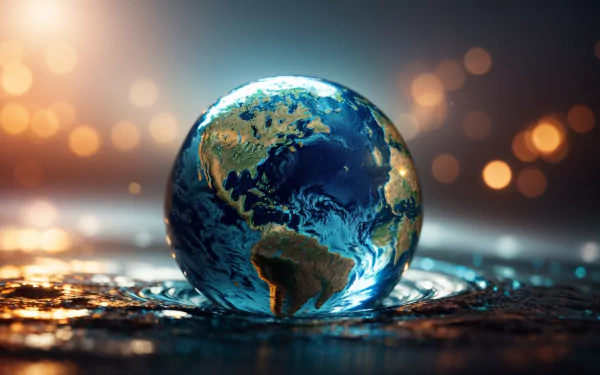
JointDiT:EnhancingRGB-DepthJointModelingwithDiffusionTransformers
We present JointDiT, a diffusion transformer that models the joint distribution of RGB and depth. By leveraging the architectural benefit and outstanding image prior of the state-of-the-art diffusion transformer, JointDiT not only generates high-fidelity images but also produces geometrically plausible and accurate depth maps. This solid joint distribution modeling is achieved through two simple yet effective techniques that we propose, i.e., adaptive scheduling weights, which depend on the noise levels of each modality, and the unbalanced timestep sampling strategy. With these techniques, we train our model across all noise levels for each modality, enabling JointDiT to naturally handle various combinatorial generation tasks, including joint generation, depth estimation, and depth-conditioned image generation by simply controlling the timestep of each branch. JointDiT demonstrates outstanding joint generation performance. Furthermore, it achieves comparable results in depth estimation and depth-conditioned image generation, suggesting that joint distribution modeling can serve as a replaceable alternative to conditional generation. The project page is available at this https URL.
相关推荐