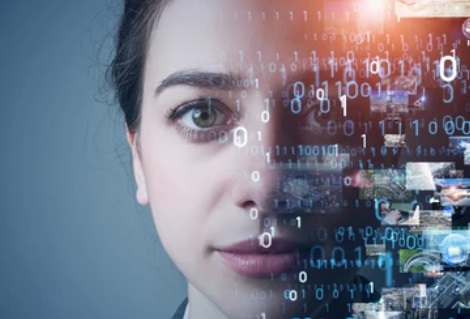
SelectingtheRightLLMforeGovExplanations
The perceived quality of the explanations accompanying e-government services is key to gaining trust in these institutions, consequently amplifying further usage of these services. Recent advances in generative AI, and concretely in Large Language Models (LLMs) allow the automation of such content articulations, eliciting explanations' interpretability and fidelity, and more generally, adapting content to various audiences. However, selecting the right LLM type for this has become a non-trivial task for e-government service providers. In this work, we adapted a previously developed scale to assist with this selection, providing a systematic approach for the comparative analysis of the perceived quality of explanations generated by various LLMs. We further demonstrated its applicability through the tax-return process, using it as an exemplar use case that could benefit from employing an LLM to generate explanations about tax refund decisions. This was attained through a user study with 128 survey respondents who were asked to rate different versions of LLM-generated explanations about tax refund decisions, providing a methodological basis for selecting the most appropriate LLM. Recognizing the practical challenges of conducting such a survey, we also began exploring the automation of this process by attempting to replicate human feedback using a selection of cutting-edge predictive techniques.
相关推荐