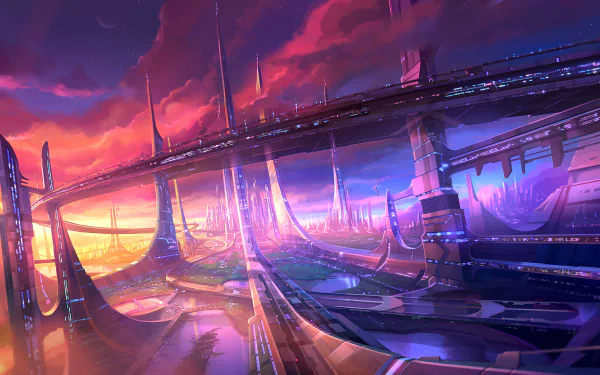
Derivativelearningoftensorialquantities--Predictingfinitetemperatureinfraredspectrafromfirstprinciples
We develop a strategy that integrates machine learning and first-principles calculations to achieve technical accurate predictions of infrared spectra. Specifically, the methodology allows to predict infrared spectra for complex systems at finite temperatures. The method's effectiveness is demonstrated in challenging scenarios, such as the analysis of water and the organic-inorganic halide perovskite MAPbI$_{3}$, where our results consistently align with experimental data. A distinctive feature of the methodology is the incorporation of derivative learning, which proves indispensable for obtaining accurate polarization data in bulk materials and facilitates the training of a machine learning surrogate model of the polarization adapted to rotational and translational symmetries. We achieve polarisation prediction accuracies of about 1 % by training only on the predicted Born effective charges.
相关推荐
热门文章
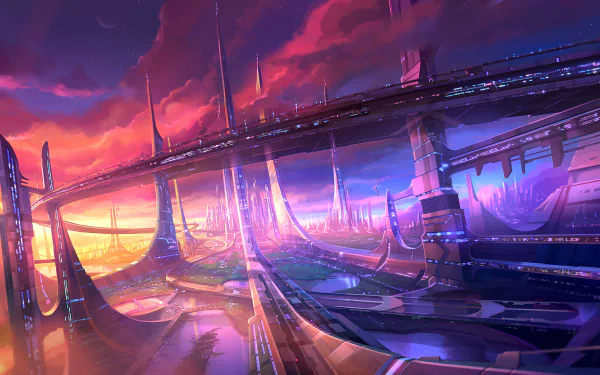