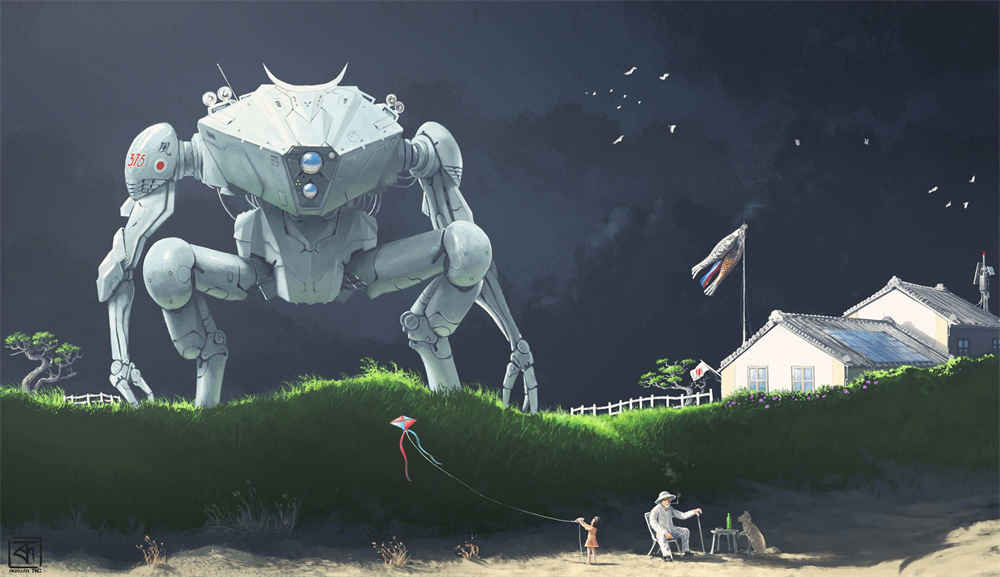
RefinedBoundsonNearOptimalityFiniteWindowPoliciesinPOMDPsandTheirReinforcementLearning
Finding optimal policies for Partially Observable Markov Decision Processes (POMDPs) is challenging due to their uncountable state spaces when transformed into fully observable Markov Decision Processes (MDPs) using belief states. Traditional methods such as dynamic programming or policy iteration are difficult to apply in this context, necessitating the use of approximation methods on belief states or other techniques. Recently, in (Journal of Machine Learning Research, vol. 23, pp. 1-46, 2022) and (Mathematics of Operations Research, vol. 48, pp. 2066-2093, Nov. 2023), it was shown that sliding finite window based policies are near-optimal for POMDPs with standard Borel valued hidden state spaces, and can be learned via reinforcement learning, with error bounds explicitly dependent on a uniform filter stability term involving total variation in expectation and sample path-wise, respectively. In this paper, we refine these performance bounds and (i) extend them to bounds via uniform filter stability in expected Wasserstein distance leading to an error bound in expectation, and (ii) complementary conditions bounds via uniform filter stability in sample path-wise total variation distance leading to a uniform error bound. We present explicit examples. Our approach thus provides complementary and more refined bounds on the error terms in both total variation and Wasserstein metrics, offering more relaxed and stronger bounds over the approximation error in POMDP solutions on the performance and near optimality of sliding finite window control policies.
相关推荐